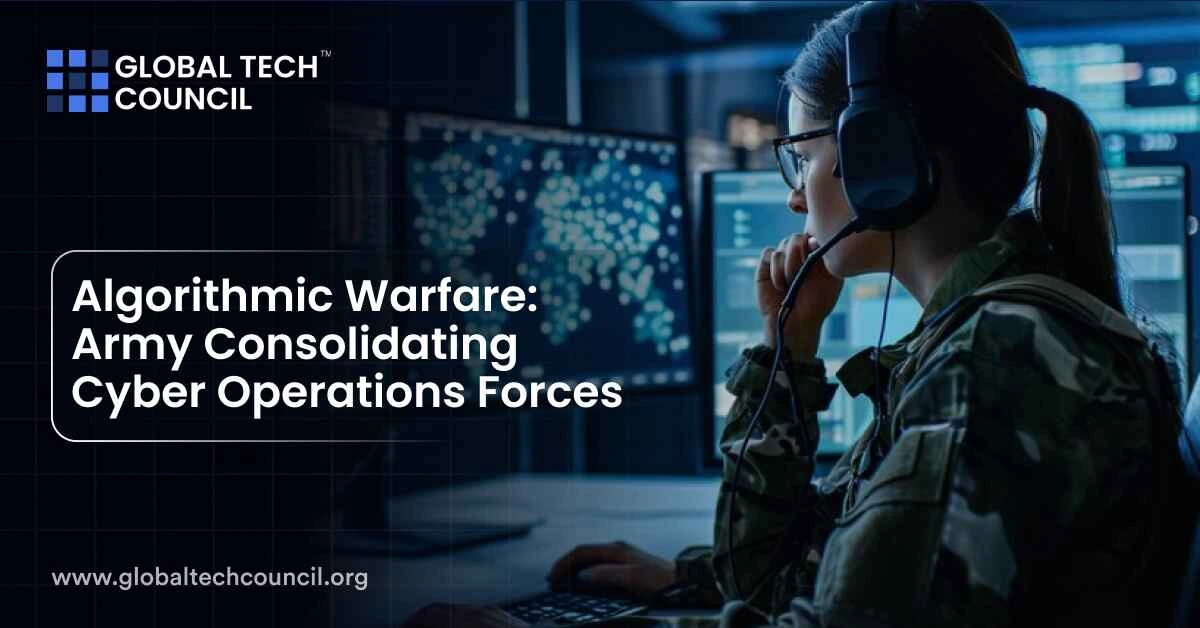
Conflict may be lost or won based on strategic balances, or means by which units of protection will asymmetrically fight the adversarial benefits. Conventional equivalents are also supplemented by artificial intelligence (AI) with great-power competitiveness, adversarial technology overmatch, and ever-expanding theatres. However, the potential of the Defense Department and the artificial intelligence experts to operationalize it is developing. The Pentagon’s initial AI programs concentrate on moving commercial technologies to the defense sector, emphasizing technological excellence and underlining mission-oriented operation.
Blog contents
-
- Introduction to Algorithmic Warface
- Operationalizing Artificial Intelligence
- Combining Technical Methodologies and Defense Doctrine
- A Framework to Operationalize Artificial Intelligence for Defense
- Measuring Effectiveness
- Effectiveness Description – A Technical Wave Top
Some Advantages of Algorithmic Warface - The transition of Artificial Intelligence to the Real World
- Conclusion
Let’s take a closer look at how Artificial Intelligence is transforming warfare.
Introduction to Algorithmic Warface
Currently, the term “algorithmic warfare” combines technical and military features. Algorithmic warfare aims to reduce the number of warfare operators in harmful ways, increase decision speed in time-critical operations, and operate where and when humans are unable to operate. However, none of these goals speak to mathematics or computer science; they are squarely grounded in military-end states. The problem is that the bridge was never established between the disciplines of science, technology, engineering, and mathematics, and the military end states before the Pentagon embarked on its AI trajectory.
Operationalizing Artificial Intelligence
Operationalization relies on the assumption that AI is not an end-state, but rather one way to achieve a military benefit. To that end, the OE needs to combine the technological execution of AI-related methodologies. This approach varies from conventional thinking since AI developers‘ strategies are usually designed to meet a specific statistical criterion (e.g., recall, accuracy), rather than a military purpose.
The result is algorithmic warfare based not only on statistics but also on a broader tactical significance architecture. Five criteria couch their relevance:
- Strong Profitability,
- Adaptability to existing and expected conditions,
- Insight prioritization over details,
- The degree of autonomy needed for operation, and
- Readiness on the field.
Combining Technical Methodologies and Defense Doctrine
Developing efficiency measures (EMAs) for military AI systems involves translating analysis and technological methodologies (e.g., grounded theory) into DOD doctrine. Without this mapping, algorithmic warfare is reduced to creating algorithms rather than the operational deployment process. For instance, a computer vision algorithm created to identify objects in a video is reduced to the number of vehicles that the model identifies, or how accurately those vehicles are detected. Quality, then, is what the algorithm correctly considers 85% of the time for vehicles.
A Framework to Operationalize Artificial Intelligence for Defense
As stated earlier, operationalized five aspects of mission utility define AI: minimum viability, ability to adapt to unknown and unknowable scenarios, prioritization of insight over information, required level of application autonomy, and readiness for the battlefield. The algorithmic warfare is fundamental to each of these MOEs. Analysis of this data results in a comprehensive indicator and effect framework for each of those MOEs. Doctrinal definitions and procedures underpin the entire framework.
Measuring Effectiveness
A go-no-go, the top-down system depends on the military method for calculating effectiveness. This implies a measure only exists because there is indeed every single predictor of that measure. Similarly, an indicator is only present if there are indeed all results of that indicator. It is a simple, all-or-nothing mechanism that is as readily applicable to AI as a traditional military operation.
Although traditional and AI MOEs vary in tactical execution, the underlying mechanism for validating decision-making is the same. Mainly if there is an explicit knowledge of the AI domain, can AI MOEs be validated, much in the same way that fighting weapons could not verify mOEs produced by the intel branch.
Effectiveness Description – A Technical Wave Top
Algorithmic warfare is fighting warfare utilizing artificially intelligent devices. Artificially intelligent means are those who are not only intelligent (gathering and applying insight) but also artificial (acting on knowledge in a way that humans can not). Systems must learn how to interpret data for themselves without human interference. For this, another concept is called machine learning. There are various forms of machine learning, but unsupervised machine learning will become the gold standard when it comes to the battlefield due to its simplicity and ability to extract outputs from unknown and unstructured knowledge.
Some Advantages of Algorithmic Warface
- System Flexible and Adaptability: Remember that unsupervised algorithms, due to their flexibility and ability to derive insight even in new scenarios, are ideal for live missions. In short, without predetermined information, unsupervised systems can operate and learn as new information becomes available. Successful algorithmic warfare programs will require the same adaptability of service members to their tactical execution and learning ability.
- Reducing Mission Complexity: Deep learning is known to reduce complexity. The reduction of complexity in a live mission is about the way knowledge is interpreted and understood. Successful algorithmic warfare, as with humans, is based on pattern recognition, reasoning, and problem-solving.
- Operating with Little to No Guidance: Given that algorithmic warfare implies that other than human means are leveraged, AI must independently write and adjudicate course of action. AI must complete the adjudication based on its decision-making, flexibility, and knowledge of circumstances.
The transition of Artificial Intelligence to the Real World
Battlefield readiness is a measure of whether the system is capable of functioning in live spaces. Since there are vast mission constraints, AI can not be developed in a laboratory without preconceiving how it will operate in the actual world. To be clear, the battlefield doesn’t circumvent the limitations of laboratory AI; they’re amplified. Military infrastructure restrains open architectures. Siloed, legacy systems bogged the Agnostic pipelines. Once deployed forward, pervasive, high-speed networking becomes sporadic or intermittent. And universal commercial-sector uncleared experts are replaced by access-limited user communities with certified artificial intelligence experts.
Conclusion
Operationalizing AI is an inherently mission-centric undertaking that has to make tactical sense for any strategic effect. Unless there is a meaningful return on investment for units on the field, widespread uncertainty will remain about the importance of algorithmic warfare; thus, an increasingly unwinnable situation will become adversarial overmatch. Without a system to operationalize such projects, the DOD can not continue implementing AI programs. Artificial intelligence training can play a vital role in establishing the pillars of algorithmic warfare.
References
https://www.armyupress.army.mil/Journals/Military-Review/English-Edition-Archives/July-August-2020/Crosby-Operationalizing-AI/