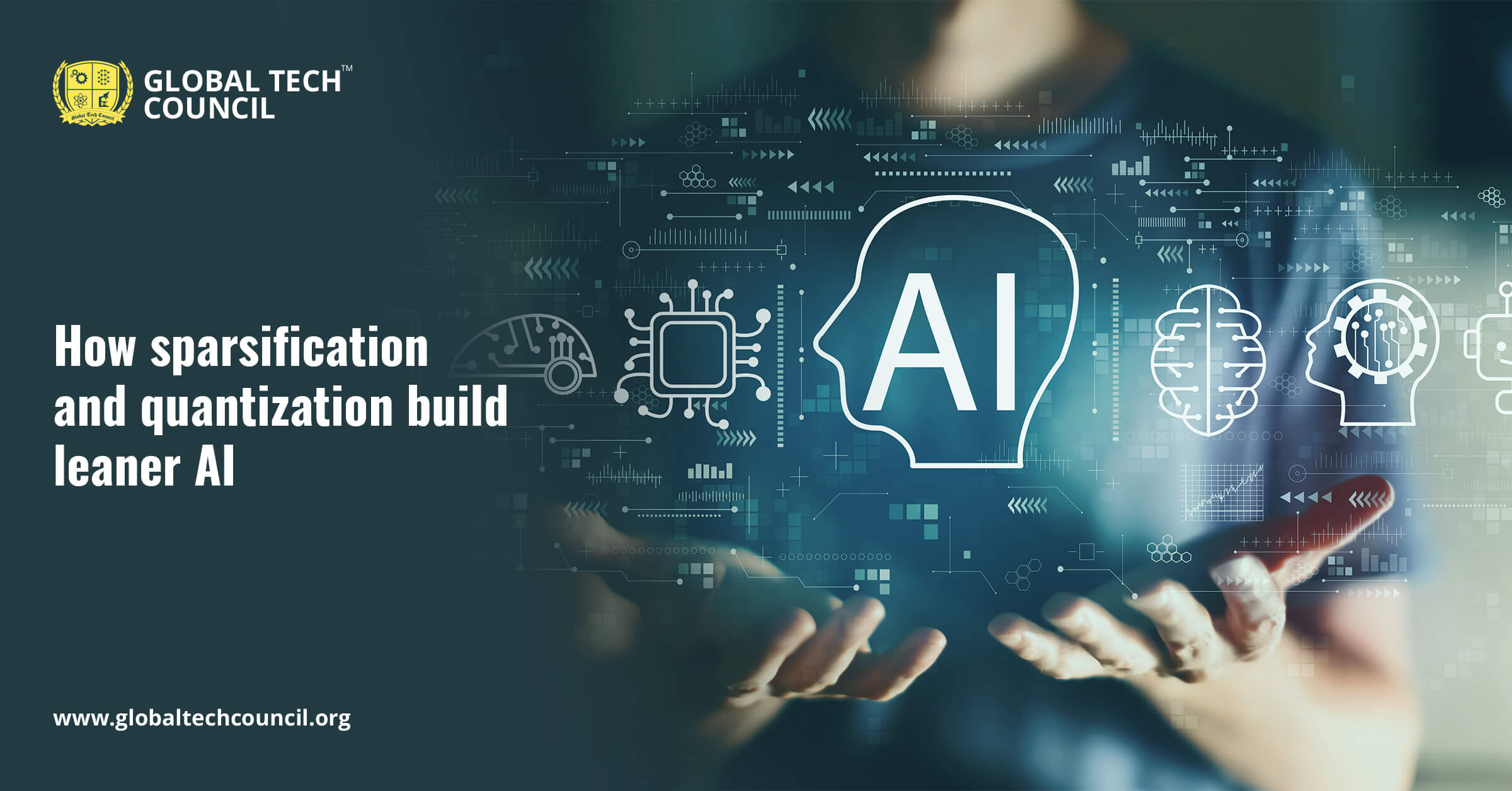
Artificial Intelligence or AI and Machine Learning or ML are topics that seldom go out of style. Technology providers are vying for the top spot in the artificial intelligence and machine learning industry. They are eager to demonstrate how their methodology to automation can help with everything from predicting upkeep for machinery and equipment to indicating when meatless sausages will be ordered online shopping purchases.
Much of the argument about artificial intelligence revolves all-around software applications that tech companies develop as a response to it. Suppose you would like to learn something about how the “explainable” artificial intelligence functions work, as well as what they can accomplish for us. The requirement to guarantee that human insensible or possibly semi-conscious thought is not encoded into the developing technologies is a critical component of that interpretability.
A procedure that uses much energy
The energy-intensive procedure of learning and implementing the artificial intelligence engines that operate inside these systems has slowed specific artificial intelligence and machine learning progress. This has prompted some companies to reconsider how they obtain energy to run their plans, with AWS, for example, seeing the entire cloud AI ecosystem as a flag-waving activity as well as of itself.
Several industry observers believe that with every breakthrough in artificial intelligence technology comes an incremental growth in the power required to train and operate the AI, thus increasing the environmental effect. Dr. Eli David is the co-founder and CTO of DeepMind, which is based in Tel Aviv. David has concentrated his effort on enhancing deep learning using additional software as a self-proclaimed academic expert in a deep understanding and neural networks.
DeepCube is a software-oriented inference accelerator that may reorganize deep learning systems during their training process. It can be installed on top of the current hardware. Early findings reveal a tenfold reduction in overall size, lowering the computing power required to execute the system in real-world scenarios.
Sparsification is a process that cleans up neural brain patterns.
DeepCube’s patented technology, according to Dr. David and his colleagues, is modeled after the human brain, which experiences trimming throughout its early training phase. Therefore, it is most receptive to sparsification, or the process of making something much smaller.
Like the early phases of human brain growth, the deep learning model receives a massive volume of information in its initial stages. However, as training progresses, neuronal connections get more robust and alter to enable ongoing learning. Layoffs are generated as each link grows more potent. Therefore conflicting linkages can be eliminated. This is why, according to David, continual restructuring and sparsification of deep learning models throughout training or rather than after training is required.
The DeepMind software development team notes that even after the artificial intelligence training step, the AI model has wasted a substantial percentage of its ‘plasticity.’ This implies that brain connections can’t adjust to take on more responsibilities, which indicates that eliminating them might lead to lower accuracy. Throughout the training, the machinery that will execute the system is etched to ensure that optimization is particular and optimized rather than naive and general. Low power and energy budgets are consumed for each machine learning job as a result of this procedure.
Prudent pruning boosts AI performance.
Current approaches, in which efforts are made to reduce the size of the deep learning model after training, have had some effectiveness. Ai developer may dramatically enhance outcomes if you prune early in the training phase when the system is most susceptible to rebuilding and adjusting. When sparsification is done while training, the links are still in the fast learning phase and can be programmed to take over the activities of the links that have been deleted, as per David. As a result, the resultant model AI might be compact, with many performances as well as memory reductions, allowing for effective utilization on intelligent edge equipment like drones, agricultural machines, mobile devices, as well as preventative maintenance.
According to David and his colleagues, this technique can be critical to make gadgets bright while decreasing their environmental effect and enabling robots to make genuinely independent judgments without rising global temperatures. The usage of such sparsification enables Ai certification technologies to function with less energy usage and, in principle, less environmental effect by shrinking the model’s dimensions by about 85-90 percent on average and boosting the performance by ten times. This technique also enables more artificial intelligence to be implemented in a relatively smaller processing footprint, which is excellent news for Internet of Things (IoT) ‘edge’ gadgets required to be more innovative.
Conclusion
The above-mentioned entire conversation speaks to a deeper substrate of future innovation that many of us would not think of as the most important or pressing problem in AI today. However, as we’ve seen previously, it’s often the ‘essential aspect’ that defines an item or solution what it is. Even so, microprocessor speeds were at the heart of the PC revolutions.