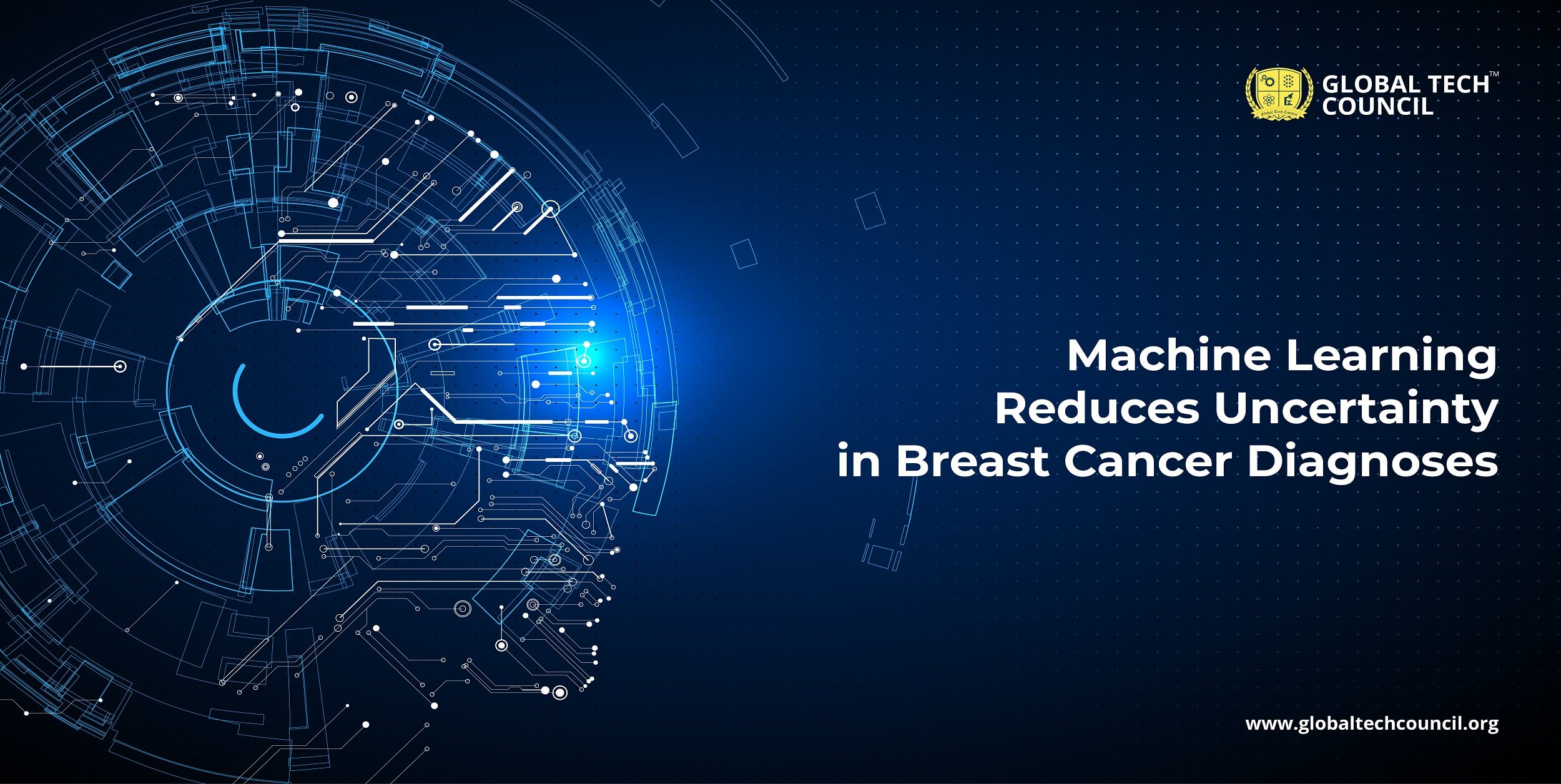
A machine-learning algorithm created at Michigan Tech utilizes probability to diagnose breast cancer in histopathology pictures better and assess the uncertainty of its predictions. The most frequent cancer with the most excellent fatality rate is breast cancer. Rapid discovery and diagnosis reduce the disease’s impact however, due to data bias and the lack of annotated data in significant amounts, identifying breast cancer using histopathology photos, tissues, and cells inspected under a microscope is a complex process. The use of a convolutional neural network (CNN), a machine learning approach, to identify breast cancer automatically has shown promise; nonetheless, it is coupled with a significant risk of false positives and false negatives.
CNN’s erroneous forecasts might have disastrous consequences if there is no way of knowing how accurate they are. Meanwhile, a new machine-learning algorithm created by researchers at Michigan Technological University can assess the uncertainty in its predictions as it diagnoses benign and malignant tumors, thereby lowering the danger.
Mechanical engineering graduate students Ponkrshnan Thiagarajan and Pushkar Khairnar and Susanta Ghosh, assistant mechanical engineering and machine learning certification outline their novel probabilistic machine learning online courses, which outperforms comparable models, in a paper recently published in the journal IEEE Transactions on Medical Imaging.
“Any machine learning technology that has been built up to this point would have some uncertainty in its prediction,” Thiagarajan explained. “Quantifying that uncertainty is difficult.” We don’t know the level of confidence in an algorithm’s prediction that a person has cancer.”
Confidence comes from experience
It isn’t easy to trust computer-generated forecasts in medicine since we don’t know how confident an algorithm is. The current model is a variant of the Bayesian neural network, a machine learning certification that can assess a picture and generate a result. This model’s parameters are represented as random variables to simplify uncertainty quantification.
The Michigan Tech approach distinguishes between negative and positive classes by examining pictures, which are nothing more than collections of pixels at their most fundamental level. The model may also quantify the uncertainty in its predictions and this categorization.
A model like this claims to save time in a medical laboratory by categorizing photos faster than a lab tech. In addition, because the model can assess its degree of confidence, it may refer photos to a human expert when it is unsure.
But why is machine learning developing medical algorithms? His idea germinated when Thiagarajan began utilizing machine learning to lower the computing time required for mechanical engineering issues. If a calculation analyses the deformation of construction materials or decides whether a person has breast cancer, it’s critical to understand the uncertainty of that computation.
“One of the malignancies with the highest mortality and incidence in breast cancer,” Thiagarajan added. “We think this is a fascinating subject where better algorithms can have a direct influence on people’s lives.”
Steps to Follow:
The researchers plan to expand the algorithm for multiclass categorization of breast cancer now that their work has been published. In addition to identifying benign and malignant tissues, their goal will be to discover cancer subtypes. The model, built using histopathological scans of breast cancer, can also be used for another medical diagnosis.
The most frequent cancer with the most excellent fatality rate is breast cancer. Early discovery and diagnosis can help to lessen the disease’s impact. Furthermore, identifying breast cancer using histopathological pictures is challenging due to data bias and the lack of annotated data in sufficient numbers.
Using a convolutional neural network (CNN) to identify breast cancer has shown potential automatically. However, it is associated with a significant risk of false positives and negatives.
“Despite the promise of machine learning-based classification models, their predictions are subject to uncertainty due to intrinsic randomness and bias in data, as well as the paucity of big datasets,” Ghosh explained. “Our study, our work attempts to address these issues and quantifies, uses and explains the uncertainty.”
Finally, Thiagarajan, Khairnar, and Ghosh’s model, which can assess whether pictures have high or low levels of ambiguity and determine whether images require the assistance of a medical professional, is the next stage in the machine learning online courses journey.
According to Thiagarajan, breast cancer is one of the malignancies with the most extraordinary fatality and incidence rates. “We think this is a fascinating subject where better algorithms can have a direct influence on people’s lives.”
What was the outcome?
By studying the photographs, which are collections of pixels at their most basic level, the Michigan Tech model distinguishes between negative and positive teachings. The design can also assess the degree of uncertainty in its predictions and this categorization.
This gadget offers time savings in a medical laboratory by identifying photos faster than a lab worker. Furthermore, because the model can assess its degree of conviction, it may submit the photographs to a human specialist if it is unsure.
Moving forward, the researchers intend to develop the model for multiclass categorization of breast cancer. In addition to identifying benign and malignant tissue, their purpose is to discover cancer subtypes. Other medical diagnostics might be produced using the machine learning technology approach.
Statement of the Issue
The UCI Machine Learning Repository’s breast cancer database is a freely accessible dataset. It details tumor characteristics such as size, density, and texture. To develop a classification model that uses numerous factors to predict whether a cancer diagnosis is benign or malignant.