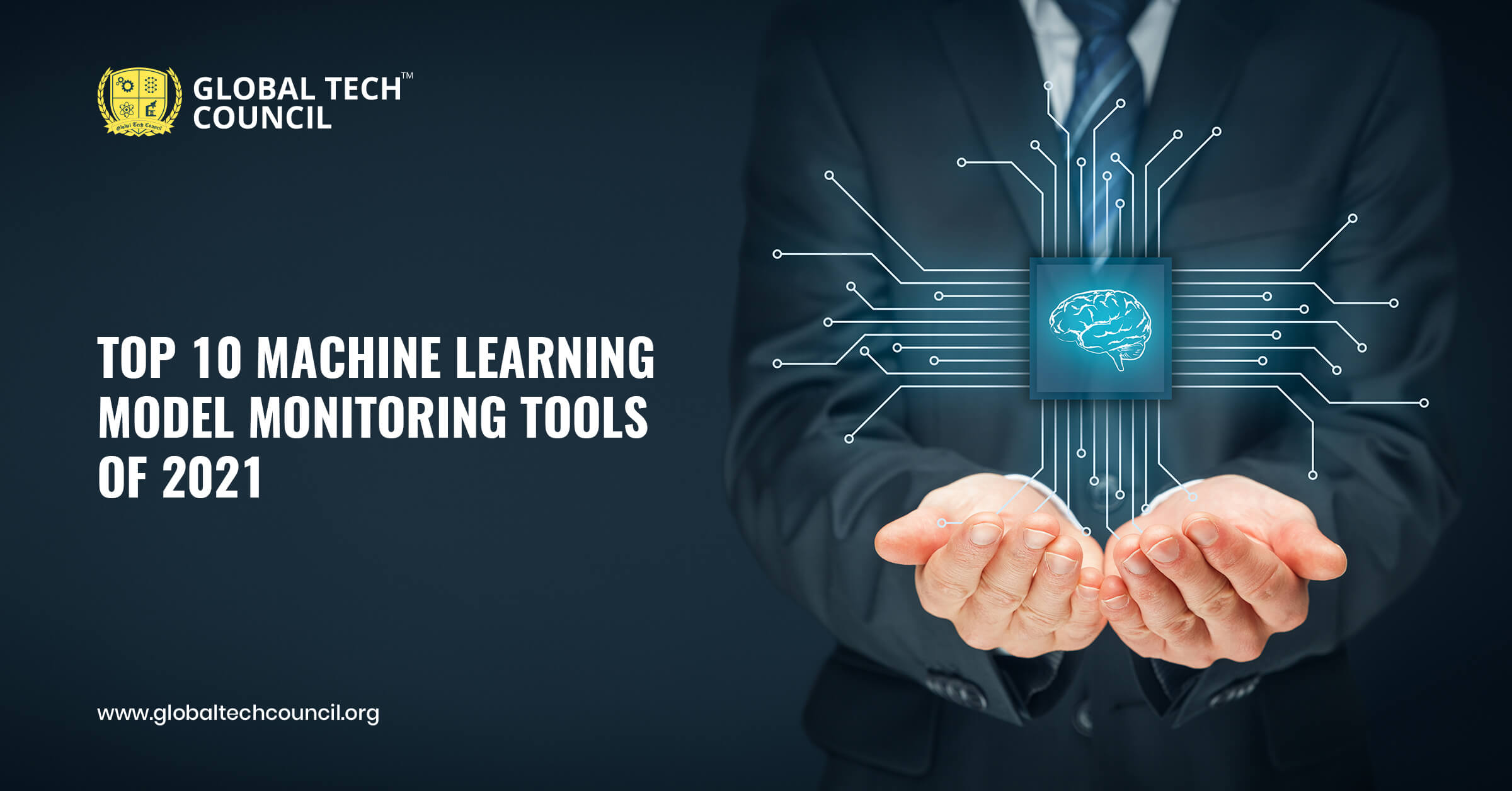
Machine learning is becoming more critical and necessary technology day by day. It helps the machines to learn things and grow their intelligence capability. Many fields like artificial intelligence, data science, automation use the technology of ML. The scope and spread of machine learning are expanding like never before. It has many learning models, and monitoring tools as machine learning is a tremendous usable technology, so it is essential to know the monitoring tools.
10 Machine Learning Model Monitoring Tools of 2021:
As of 2021, the use of machine learning is increasing. Machine Learning is highly efficient for business growth models. Machine learning helps to analyze data and find valuable insights. These insights help to generate leads and help to grow the profit of the business. Many organizations are also reliant on machine learning models and monitoring tools. The machine learning monitoring tools help to make animation and unsupervised learning algorithms. It also helps to nullify the prediction error and data visualization. The expert expects that the machine learning monitoring tool can grow by $4 billion shortly.
Here we briefly discuss the ten best machine learning monitoring models to use. The tools are,
- Anodot:
Anodot is a popular and beneficial machine learning monitoring tool. It is essential to properly use a considerable amount of data for the growth of the business. Anodot is a tool that can help to handle the data easily. It is artificial intelligence or an AI motoring tool. It helps to understand the information automatically. Anaconda also enables the user to monitor multiple things simultaneously, and Anodot helps to handle the vast databases, like, customer experience, revenue, or customer details.
- Google Cloud AI platform:
The platform is a well-known and popular platform with superb usability. Google cloud AI gives a comprehensive and reliable experience to the user. However, the platform offers both code or no code, both types of machine learning experiences. The venue is perfect for hybrid cloud deployment. It provides 25 parallel running models, point and clicks data science autoML, and model optimization.
Furthermore, Google’s platform has great video processing modules, and it connects the TPUs and TensorFlow. It also combines the autoML, MLOps, and AI platforms. This makes the platform a great one to work with.
- Flyte:
It is an open-source MLOps platform. Flyte helps to maintain the data, monitor and track the data. It mainly focuses on monitoring the changes of the model, and it also makes sure that the model is reproducible. Written in Python coding language, Flyte can support Java, Scala, and Python. Furthermore, the platform helps to keep the organization compliant with any kind of data change. The intelligent use of the cache output of Flyte helps to save time and cost. Flyte platform offers easy data handling preparation, model training. It also provides metrics computation, data validation, and visualization.
- Anaconda:
Anaconda is a widely used platform for machine learning. It is not only a simple machine learning monitoring tool, but it also has many useful functions to use. The platform offers many valuable libraries and different python versions. It offers pre-installation of any other libraries and packages. Furthermore, it is a multi-problem solver tool that can solve different types of problems in no time and has 7500 Conda packages and costs only $14.95. It also comes with a free edition.
- TensorFlow:
TensorFlow is the best tool for people who are more comfortable with mobile phones and prefer to work with mobile phones. This free tool offers huge libraries of different functions. It also provides debug and training processes with varying parts of images, text, and video. The platform also provides a deep learning framework.
- ZenML:
It is the best tool to check the compatibility between 2 experiments. Furthermore, it offers built-in extraction of training jobs, model serving and can even effectively reproduce through auto-track experiments, version code, and data. It is compatible with the Jupyter notebook and is best for evaluating data sets.
- Seldon Core:
It enables the user to deploy machine learning models, and manage, monitor, and package multiple models. It can run on any cloud with a robust security system. Furthermore, it can convert Java, Python, or ML models into REST or GRPC production microservice.
- Fiddler:
This user-friendly tool is one of the best for ML model monitoring. It gives an easy interface for debugging prediction, explaining or analyzing the model behavior, and managing databases.
- Pachyderm:
It is a free Ml tool that can analyze vast data of companies. Furthermore, the platform helps to make repeatable and scalable experiments. So, many organizations like the woven planet, digital reasoning, or general fusion use the tool. The platform has Kubernetes and dockers foundation, and it helps to deploy ML models on different clouds.
- KFServing:
This monitoring tool further provides Tensiorflow, XGBoost, PyTorch, Performant, ONNX, abstract interface. All these help to solve production model serving. Furthermore, it is compatible with Kubeflow 1.3 and is used to make the deployment scalable and straightforward.
Conclusion:
So these were the top 10 machine learning model monitoring tools of 20201. The popularity, usability, and efficiency of machine learning are increasing exponentially. Machine learning courses and machine learning certification are also growing. Machine learning online paths give easy online certificates to the students. The accreditation can land one in a great machine learning career. It is also essential to learn the monitoring tools to work more efficiently and quickly in the ML field.
Leave a Reply